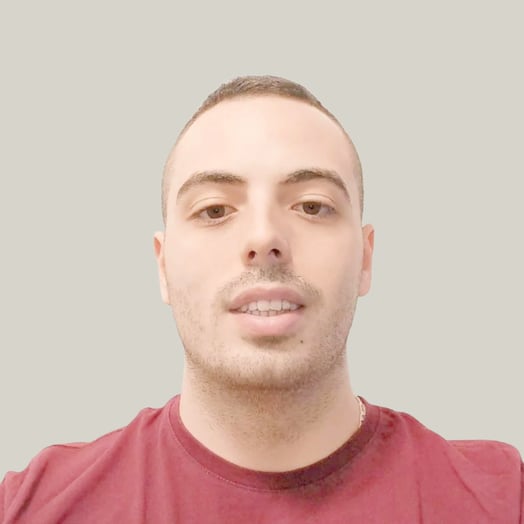
Stefan Mićić
Verified Expert in Engineering
Python数据工程师和开发人员
Stefan is an experienced machine learning and machine learning operations (MLOps) engineer with hands-on experience in big data systems. His demi-decade of expertise is supplemented by a master's degree in artificial intelligence. Stefan研究过物体检测等问题, classification, sentiment analysis, 命名实体识别, 推荐系统. He is always looking forward to being involved in end-to-end machine learning projects.
Portfolio
Experience
Availability
Preferred Environment
PyCharm, Python 3, Python, GitHub, Amazon S3 (AWS S3), JSON, Distributed Systems
The most amazing...
...end-to-end machine learning solution I've created optimized the cost of the machine learning pipelines numerous times with state-of-the-art results.
Work Experience
Machine Learning Engineer
RhythmScience Inc.
- 确定数据库和各种类型的文件(HL7), XML, and PDF) by HIPAA standards and dockerized and automated the whole pipeline.
- 开发ML算法生成文本和分类PDF报告.
- 设计、实现和部署解决方案.
Senior MLOps Engineer
PlusPower
- 使用Sagemaker开发大型ML管道,包括预处理, training, evaluation and deployment.
- Developed pipeline that was able to generate airflow pipelines based on configs and automated deployment of DAGs.
- Increased test coverage from 15% to 80% and added integration tests so that we can test sagemaker pipelines locally.
AI Lead via Toptal
Cumulus Technologies LLC
- 在AWS上创建了整个CI/CD管道. Everything from data ingestion, processing, and model training to model deployment was automated.
- Designed and led the implementation of the whole ML pipeline using various AWS services such as Lambda, Polly, and SageMaker.
- Utilized AWS for development to meet high security requirements (AWS Cloud9, AWS CodeCommit, and AWS CodePipeline).
MLOps Engineer
NewsCorp
- 执行不同LLM和稳定扩散模型的部署.
- 致力于llm的延迟和成本优化. Successfully reduced latency by five times using different deployment techniques.
- Took responsibility for the complete deployment process of the whole ML part and documentation maintenance.
MLOps Engineer
PepsiCo Global - DPS
- Implemented an end-to-end pipeline using PySpark machine learning pipeline.
- 使用GitHub操作实现了单元和集成测试的CI/CD.
- Implemented Spark and scikit-learn/Pandas ETL jobs for handling large volumes of data (150 TB).
Tech Lead Data Engineer
Motius
- Led a small team in implementing an ELT pipeline to get data from a GraphQL database and put it into Azure SQL. 所有内容都被Dockerized并推送到Azure映像注册表.
- Implemented KPI calculations using PySpark, which was communicating with Snowflake. 为Snowflake定义了表模式,并创建了迁移脚本.
- Followed the Scrum methodology, including daily scrums, retro, and planning, and used Jira.
- Led a small team in implementing ETL Spark jobs with Apache Airflow as an orchestrator, AWS是基础设施,Snowflake是数据仓库.
MLOps Engineer
Lifebit
- 使用量化进行深度学习模型优化, ONNX Runtime, and pruning, among others.
- 监控模型性能,包括内存、延迟和CPU使用情况.
- Used Valohai to automate the CI/CD process and GitHub Actions to automate some parts of the MLOps lifecycle.
- 使用Amazon CloudWatch创建了自动实验跟踪, Valohai, Python, GitHub Actions, and Kubernetes.
Machine Learning Engineer
HTEC Group
- Optimized a machine learning compiler already on a trained network without re-training using 开放神经网络交换(ONNX) and implemented custom operators using PyTorch and C++.
- Worked on an Android machine learning solution and mentored a less experienced developer to train and prepare an object detector and classifier to run smoothly on an Android device.
- Enhanced a project that aimed to upscale images to be as perfect as possible toward 4K resolution.
- 参与船舶路线的SDP问题. 从零开始实现了一个算法来引导船只. 油耗和预计到达时间被用于计算.
- Worked on open source ONNX Runtime in order to add support for the MIGraphX library.
Machine Learning Engineer
SmartCat
- 使用MLflow进行模型版本控制,为完成MLOps生命周期做出贡献, 用于数据版本控制的LakeFS, AWS S3 for data storage, 和TensorFlow在Docker中服务.
- Functioned as a data engineer using Apache Spark for ETL jobs with Prefect and Apache Airflow for scheduling.
- Trained several different architectures for object detection and classification.
Machine Learning Engineer
Freelance
- 从各个网站搜集欧博体育app下载, then analyzed and prepared the scraped data for web shops using natural language processing—long short-term memory (LSTM), Word2Vec, 和转换器——因为数据是塞尔维亚语,所以添加了NER.
- Used Amazon SageMaker to automate the machine learning pipeline—data preprocessing, model training, and deployment. 执行模型的自动再培训和部署, 在客户端更新新数据之前完成机器学习过程.
- Worked on big data projects using Apache Spark, Kafka, Hadoop, and MongoDB.
- 作为数据工程师,使用Spark创建优化的ETL管道. 将客户的需求转换为SQL.
Experience
自动化端到端(E2E)计算机视觉解决方案
•检测房间中的物体
•分类人的姿势
•自动再培训(主动学习)
•模型和数据版本控制
• Dockerized pipeline
利用这些模型和预测, we created a post-processing pipeline for creating reports or key performance indicators (KPIs) for clients.
Android COVID-19测试分类
我在这个项目上领导了一个两个人的团队. We used MobileNet due to size, and all business-relevant metrics were great. 我们使用了许多优化技术将模型部署到Android上, such as quantization, pruning, 知识的提炼.
MLOps Engineer
Image Super Resolution
ETL Jobs
•优化解决方案,降低成本和计算时间.
•通过气流和Prefect计划作业.
The tech stack was: Spark, Scala, AWS S3, Kafka, Apache Airflow, and Prefect.
NLP Articles Processing
1. 找到所有相关的标签(事件、地点、名称等).) in the article.
2. 找到在某种程度上相关的标签对.
Hugging Face transformers were mainly used to tackle this problem (BERT-based models). 总体指标高于95%.
Data Ingestion
DE项目的技术领导
Education
人工智能硕士学位
诺维萨德大学-诺维萨德,塞尔维亚
Certifications
AWS认证机器学习-专业
Amazon Web Services
Skills
Libraries/APIs
PyTorch, Keras, NumPy, Scikit-learn, REST APIs, TensorFlow, Pandas, PySpark, Terragrunt
Tools
PyCharm, Amazon SageMaker, GitHub, Apache Airflow, Pytest, Codeship, AWS Glue, Bitbucket, Grafana, Terraform, Celery
Frameworks
Spark, Apache Spark, Streamlit
Languages
Python 3, Python, SQL, Scala, Java, Snowflake, GraphQL, c++
Paradigms
数据科学,ETL,单元测试,DevOps
Platforms
Amazon Web Services (AWS), Jupyter Notebook, Visual Studio Code (VS Code), Docker, Kubernetes, Amazon EC2, Apache Kafka, Azure, Databricks, 谷歌云平台(GCP), Hyperledger Fabric, Kubeflow
Storage
Amazon S3 (AWS S3), JSON, Databases, PostgreSQL, NoSQL, MongoDB, Data Pipelines, Database Migration, Data Integration, Azure SQL, Datadog
Industry Expertise
交易系统,项目管理
Other
Deep Learning, Machine Learning, 人工智能(AI), Data Engineering, Computer Vision, 自然语言处理(NLP), 自然语言理解(NLU), 卷积神经网络(CNN), 递归神经网络(rnn), 机器学习操作(MLOps), Neural Networks, AI Design, Deep Neural Networks, Software Engineering, Technical Hiring, Source Code Review, Code Review, Task Analysis, Interviewing, APIs, GPT, 生成预训练变压器(GPT), 大型语言模型(llm), Models, Data Processing, English, 生成式人工智能(GenAI), Language Models, MLflow, OpenAI, Recommendation Systems, 开放神经网络交换(ONNX), Lens Studio, Optimization, Team Leadership, Valohai, Time Series, Data Modeling, Data Mining, Monitoring, Big Data, Image Processing, Transformers, Cloud, Object Detection, 计算机视觉算法, Object Tracking, Web Development, Speech Recognition, Voice Recognition, Cloud Services, ETL Tools, Distributed Systems, Data Analysis, CI/CD Pipelines, Query Optimization, Research, Stock Trading, Algorithmic Trading, Finance, Financial Software, Prompt Engineering, 检索增强生成(RAG), OpenAI GPT-3 API, OpenAI GPT-4 API, Prefect, Data Analytics, ELT, Hugging Face, BERT, Back-end, Software Architecture, DocumentDB
How to Work with Toptal
Toptal matches you directly with global industry experts from our network in hours—not weeks or months.
Share your needs
Choose your talent
开始你的无风险人才试验
对顶尖人才的需求很大.
Start hiring